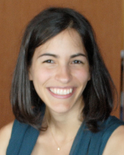
Marta Recasens
I joined Google as a Research Scientist in 2013. I work on Natural Language Processing, and in particular on various problems around linguistic reference, including coreference resolution.
I completed my PhD at the University of Barcelona in 2010 and was a postdoctoral scholar at Stanford University before joining Google. Check my personal website for more information.
Research Areas
Authored Publications
Google Publications
Other Publications
Sort By
Mind the GAP: A Balanced Corpus of Gendered Ambiguous Pronouns
Transactions of the Association for Computational Linguistics, vol. 6 (2018), pp. 605-618
Preview abstract
Coreference resolution is an important task for natural language understanding, and the resolution of ambiguous pronouns a longstanding challenge. Nonetheless, existing corpora do not capture ambiguous pronouns in sufficient volume or diversity to accurately indicate the practical utility of models. Furthermore, we find gender bias in existing corpora and systems favoring masculine entities. To address this, we present and release GAP, a gender-balanced labeled corpus of 8,908 ambiguous pronoun–name pairs sampled to provide diverse coverage of challenges posed by real-world text. We explore a range of baselines that demonstrate the complexity of the challenge, the best achieving just 66.9% F1. We show that syntactic structure and continuous neural models provide promising, complementary cues for approaching the challenge.
View details
Sense Anaphoric Pronouns: Am I One?
Zhichao Hu
Olivia Rhinehart
Proceedings of the Workshop on Coreference Resolution Beyond OntoNotes (CORBON 2016), pp. 1-6
Preview abstract
This paper focuses on identity-of-sense anaphoric relations, in which the sense is shared but not the referent. We are not restricted to the pronoun "one", the focus of the small body of previous NLP work on this phenomenon, but look at a wider range of pronouns ("that", "some", "another", etc.). We develop annotation guidelines, enrich a third of English OntoNotes with sense anaphora annotations, and shed light onto this phenomenon from a corpus-based perspective. We release the annotated data as part of the SAnaNotes corpus. We also use this corpus to develop a learning-based classifier to identify sense anaphoric uses, showing both the power and limitations of local features.
View details
Resolving Discourse-Deictic Pronouns: A Two-Stage Approach to Do It
Sujay Kumar Jauhar
Raul D. Guerra
Edgar Gonzàlez Pellicer
Proceedings of the 4th Joint Conference on Lexical and Computational Semantics (*SEM 2015), pp. 299-308
Preview abstract
Discourse deixis is a linguistic phenomenon in which pronouns have verbal or clausal, rather than nominal, antecedents. Studies have estimated that between 5% and 10% of pronouns in non-conversational data are discourse deictic. However, current coreference resolution systems ignore this phenomenon. This paper presents an automatic system for the detection and resolution of discourse-deictic pronouns. We introduce a two-step approach that first recognizes instances of discourse-deictic pronouns, and then resolves them to their verbal antecedent. Both components rely on linguistically motivated features. We evaluate the components in isolation and in combination with two state-of-the-art coreference resolvers. Results show that our system outperforms several baselines, including the only comparable discourse deixis system, and leads to small but statistically significant improvements over the full coreference resolution systems. An error analysis lays bare the need for a less strict evaluation of this task.
View details
Modeling the Lifespan of Discourse Entities with Application to Coreference Resolution
Marie-Catherine de Marneffe
Christopher Potts
Journal of Artificial Intelligence Research, vol. 52 (2015), pp. 445-475
Preview abstract
A discourse typically involves numerous entities, but few are mentioned more than once. Distinguishing those that die out after just one mention (singleton) from those that lead longer lives (coreferent) would dramatically simplify the hypothesis space for coreference resolution models, leading to increased performance. To realize these gains, we build a classifier for predicting the singleton/coreferent distinction. The model’s feature representations synthesize linguistic insights about the factors affecting discourse entity lifespans (especially negation, modality, and attitude predication) with existing results about the benefits of “surface” (part-of-speech and n-gram-based) features for coreference resolution. The model is effective in its own right, and the feature representations help to identify the anchor phrases in bridging anaphora as well. Furthermore, incorporating the model into two very different state-of-the-art coreference resolution systems, one rule-based and the other learning-based, yields significant performance improvements.
View details
An Extension of BLANC to System Mentions
Xiaoqiang Luo
Sameer Pradhan
Eduard Hovy
Proceedings of the 52nd Annual Meeting of the Association for Computational Linguistics (Short Papers) (2014), pp. 24-29
Preview abstract
BLANC is a link-based coreference evaluation metric for measuring the quality of coreference systems on gold mentions. This paper extends the original BLANC (“BLANC-gold” henceforth) to system mentions, removing the gold mention assumption. The proposed BLANC falls back seamlessly to the original one if system mentions are identical to gold mentions, and it is shown to strongly correlate with existing metrics on the 2011 and 2012 CoNLL data.
View details
Scoring Coreference Partitions of Predicted Mentions: A Reference Implementation
Sameer Pradhan
Xiaoqiang Luo
Eduard Hovy
Vincent Ng
Michael Strube
Proceedings of the 52nd Annual Meeting of the Association for Computational Linguistics (Short Papers) (2014), pp. 30-35
Preview abstract
The definitions of two coreference scoring metrics—B3 and CEAF—are underspecified with respect to predicted, as opposed to key (or gold) mentions. Several variations have been proposed that manipulate either, or both, the key and predicted mentions in order to get a one-to-one mapping. On the other hand, the metric BLANC was, until recently, limited to scoring partitions of key mentions. In this paper, we (i) argue that mention manipulation for scoring predicted mentions is unnecessary, and potentially harmful as it could produce unintuitive results; (ii) illustrate the application of all these measures to scoring predicted mentions; (iii) make available an open source, thoroughly-tested reference implementation of the main coreference evaluation measures; and (iv) rescore the results of the CoNLL-2011/2012 shared task systems with this implementation. This will help the community accurately measure and compare new end-to-end coreference resolution algorithms.
View details
No Results Found