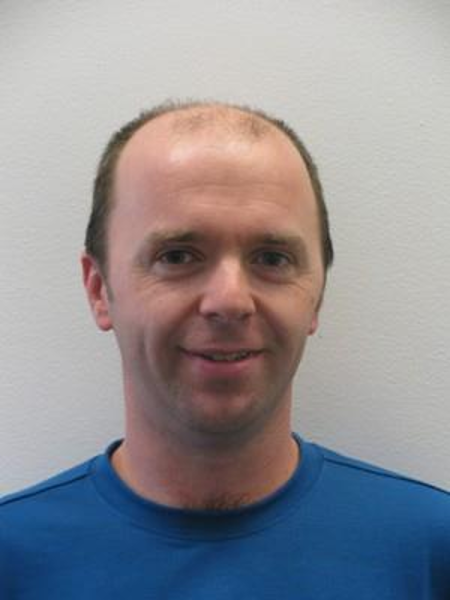
Radu Soricut
I am a Distinguished Scientist & Senior Research Director at Google DeepMind, leading teams that conduct natural language modeling and machine learning research and build large models, focusing on Multimodal (language & vision) understanding and generation. I completed my PhD at USC, where I worked with Daniel Marcu and Kevin Knight.
See also my personal webpage for more information.
See also my personal webpage for more information.
Authored Publications
Sort By
Google
ImageInWords: Unlocking Hyper-Detailed Image Descriptions
Andrew Bunner
Ranjay Krishna
(2024)
PaLI-X: On Scaling up a Multilingual Vision and Language Model
Josip Djolonga
Piotr Padlewski
Basil Mustafa
Carlos Riquelme
Sebastian Goodman
Yi Tay
Siamak Shakeri
Daniel Salz
Michael Tschannen
Hexiang (Frank) Hu
Mandar Joshi
Matthias Minderer
Filip Pavetić
Gang Li
Lucas Beyer
Anurag Arnab
Yuanzhong Xu
Keran Rong
Alexander Kolesnikov
Xiaohua Zhai
Neil Houlsby
Computer Vision and Pattern Recognition Conference (CVPR) (2024)
PaLI: A Jointly-Scaled Multilingual Language-Image Model
Piotr Padlewski
Daniel Salz
Sebastian Alexander Goodman
Basil Mustafa
Lucas Beyer
Alexander Kolesnikov
Keran Rong
Hassan Akbari
Linting Xue
James Bradbury
Chao Jia
Carlos Riquelme
Xiaohua Zhai
Neil Houlsby
International Conference on Learning Representations (ICLR) (2023)
Imagen Editor and EditBench: Advancing and Evaluating Text-Guided Image Inpainting
Su Wang
Chitwan Saharia
Shai Noy
Stefano Pellegrini
Sarah Laszlo
Mohammad Norouzi
Peter Anderson
William Chan
CVPR (2023)
MaXM: Towards Multilingual Visual Question Answering
Linting Xue
Michal Yarom
Findings of ACL: EMNLP (2023)
PreSTU: Pre-Training for Scene-Text Understanding
Jihyung Kil
Hexiang (Frank) Hu
Sebastian Goodman
Wei-Lun Chao
ICCV (2023)
Connecting Vision and Language with Video Localized Narratives
Vittorio Ferrari
IEEE / CVF Computer Vision and Pattern Recognition Conference (CVPR) 2023 (to appear)